The study of correlation between nomogram prediction of uric acid and different chemotherapy regimens in breast cancer patients
Introduction
According to the most recent data from the International Agency for Research on Cancer (IARC), the number of new cases of breast cancer (2.26 million) has surpassed lung cancer (2.21 million), making it the world’s most common cancer (1). To determine the prognosis of breast cancer and guide treatment, we currently use estrogen receptor (ER), progesterone receptor (PR), and human epidermal growth factor receptor 2 (HER2) indicators (2). However, the majority of these well-established biomarkers are either unsuitable for post-operative evaluation or are prohibitively expensive and time-consuming. Establishing treatment indicators that are easy to detect and predict outcome is still critical.
One of the most prevalent endogenous antioxidants in human blood is serum uric acid (SUA), it is effective at scavenging free radicals and may aid in the reduction of carcinogenic reactive oxygen species (ROS) (3). Higher levels of SUA, on the other hand, are damaging to the human body and can cause gout, hypertension, diabetes, kidney disease, coronary heart disease, and other conditions (4-8). SUA has been connected to breast cancer risk and prognosis in previous research (9). According to Yue and others, a high SUA is related to a higher breast cancer incidence rate and a lower survival rate (10). Tilman Kühn and coworkers, but at the other contrary, discovered that greater levels of SUA were tied to a lower incidence of breast cancer and cancer death, implying that SUA may have a barrier role in cancer (11). Given the aforementioned inconsistencies, we need to continue to examine the connection between SUA and breast cancer.
Furthermore, treatment for many solid tumors causes excessive uric acid synthesis and hyperuricemia due to the rapid disintegration of intracellular nucleic acid and enhanced purine degradation (12). The most dangerous drug-induced hyperuric acid is hyperuricemia caused by cytotoxic medicines (13). Hyperuricemia, which usually develops 48 to 72 h following cytotoxic drug treatment, can lead to tumor cytolysis syndrome (TLS), a life-threatening emergency, which should be avoided (14).
As a consequence, it is essential to keep patients’ SUA levels under control after chemotherapy. Currently, Salem and others have demonstrated that cytotoxic chemotherapy medications can increase patients’ SUA while also elucidating the mechanism behind it (12). Criscuolo and coworkers have also reached the same conclusion (13). These studies, however, do not focus on a specific type of cancer—breast cancer. As a result, the objective of this research is to explore at the clinicopathological and predictive importance of SUA levels in breast cancer patients who are taking chemotherapy, as well as to develop a related nomogram to enable clinicians better control SUA levels in advance. We present the following article in accordance with the STROBE reporting checklist (available at https://tbcr.amegroups.com/article/view/10.21037/tbcr-21-34/rc).
Methods
Patients
We retrospectively analyzed the clinical data of 206 patients with primary breast cancer admitted in the Department of breast surgery, Yunnan cancer hospital from July 2012 to June 2021, all patients were female. The average age was (48.29±8.92) years. The inclusion criteria were: all of them were diagnosed as primary breast cancer by pathological examination; the pathological data are complete and have follow-up information; serum uric acid levels were measured more than twice; received surgery in our hospital and has completed 6–8 cycles of standardized chemotherapy. The exclusion criteria were: combined with other malignant tumors; complicated with major organ (heart, liver, kidney, etc.) functional diseases; the first diagnosis was stage IV or contralateral recurrent or recurrent breast cancer; combined with diabetes, hypertension, dyslipidemia, drinking and smoking; previous radiotherapy, chemotherapy and endocrine therapy. Diagnosis and molecular typing of breast cancer according to AJCC classification (15).
Data collection
The clinicopathological data of 206 patients with breast cancer were collected, including height, weight, age, tumor size, clinical stage, lymph node metastasis, histological classification and chemotherapy regimens. Among them, 105 cases were treated with EC-T chemotherapy (epirubicin-cyclophosphamide followed by paclitaxel). Thirty cases were treated with AC-T chemotherapy (adriamycin-cyclophosphamide followed by paclitaxel), 11 cases were treated with CEF chemotherapy (cyclophosphamide + epirubicin + fluorouracil), 34 cases were treated with TEC chemotherapy (paclitaxel + epirubicin + cyclophosphamide), 26 cases were treated with THP + C-T chemotherapy. Fasting peripheral venous blood was collected from all patients before breakfast, and serum uric acid levels were measured at least twice (at the first diagnosis and during chemotherapy). The SUA concentrations collected in this study were measured by the same Hitachi automatic biochemical analyzer 7090 (Hitachi) in the laboratory department of Yunnan cancer hospital. Distant metastasis free survival time (DMFS) was defined as the time from diagnosis to distant metastasis, the total follow-up time was 80 months. In this study, we compared the changes of SUA levels in 6 different chemotherapy groups.
Statistical analyses
The best SUA cutoff value was obtained by X-tile, and the sample outcomes were divided into high SUA group and low SUA group. In order to construct nomogram, the data set is randomly divided into training set and validation set by computer, and the variables are compared. Age and weight are continuous variables. If they are normally distributed variables, conduct independent sample t-test and report them as mean ± standard deviation. Otherwise, conduct Manne-Whitney U test and report them as median and quartile range. Categorical variables were expressed as their respective percentages and analyzed by chi square test or Fisher exact test. Univariate logistic regression analysis was used to determine the factors affecting serum uric acid. At the same time, the selection of variables will be comprehensively considered with the clinical significance of each variable. All variables significantly related to serum uric acid (univariate logistic regression, P<0.06) were included in further regression analysis, that is, multivariate logistic regression analysis. In multivariate regression analysis, the variables with P<0.05, combined with the clinical significance of the variables, will be retained in the final model. Based on the results of multivariate logistic regression analysis, the nomogram was constructed by R. The score of each variable is calculated according to the value of regression coefficient. The scores of different variables are added to obtain the total score of each patient, which can be converted into the prediction probability of elevated serum uric acid. The performance of nomograms is evaluated by identification and calibration. The discrimination ability of the model is determined by the area under the receiver operating characteristic (ROC) curve, which ranges from 0.5 (no discrimination) to 1 (complete discrimination). The prediction model is calibrated by comparing the visual calibration map of the predicted probability and the actual probability that the serum uric acid is lower than the predetermined threshold. R version 4.1.2 and the IBM software SPSS version 20 (IBM Corp.) were used for all statistical analyses, and P values <0.05 were considered statistically significant.
The study was conducted in accordance with the Declaration of Helsinki (as revised in 2013). The study was approved by institutional committee board of the Third Affiliated Hospital of Kunming Medical University (No. QT202001) and informed consent was taken from all the patients.
Results
Baseline characteristics of training set and validation set
A total of 206 breast cancer patients were included in this study. Seventy percent were randomly selected into the training set (n=137), and 30% were assigned to the validation set (n=69), which was randomly assigned by computer (Figure 1). Table 1 summarizes the clinicopathological features of the training set and the validation set. There was no significant difference in pathological features between the two groups (P>0.05) (Table 1).
Table 1
Characteristics | Training cohort (n=137) | Validation cohort (n=69) | P value |
---|---|---|---|
BMI (kg/m2) | 24.839 (0.432) | 23.255 (0.473) | 0.053 |
Menopause, n (%) | 0.162 | ||
Yes | 49 (35.76) | 19 (27.53) | |
No | 88 (64.23) | 50 (72.46) | |
Age (years) | 47.57 (9.145) | 46.23 (8.955) | 0.428 |
ER, n (%) | 0.725 | ||
+ | 103 (75.18) | 50 (72.46) | |
− | 34 (24.81) | 19 (27.53) | |
PR, n (%) | 0.820 | ||
+ | 99 (72.26) | 50 (72.46) | |
− | 38 (27.73) | 19 (27.53) | |
HER2, n (%) | 0.424 | ||
+ | 39 (28.46) | 16 (23.18) | |
− | 98 (71.53) | 53 (76.81) | |
TNBC, n (%) | 0.345 | ||
+ | 20 (14.59) | 7 (10.14) | |
− | 117 (85.40) | 62 (89.85) | |
Tumor-stage, n (%) | 0.634 | ||
I–II | 103 (75.18) | 53 (76.81) | |
III–IV | 34 (24.81) | 16 (23.18) | |
Distance-metastasis, n (%) | 0.317 | ||
+ | 27 (19.70) | 18 (26.08) | |
− | 110 (80.29) | 51 (73.91) |
BMI, body mass index; ER, estrogen receptor; PR, progesterone receptor; HER2, human epidermal growth factor receptor type 2; TNBC, triple negative breast cancer.
SUA levels and chemotherapy
We did not observe a significant difference in baseline SUA levels between the 5 groups with different chemotherapy regimens (P>0.05). However, we did observe significant differences among the last chemotherapy uric acid levels, as well as among the changes from baseline levels (P<0.05, Table 2).
Table 2
Uric acid | EC-T (n=105) | AC-T (n=30) | CEF (n=11) | TEC (n=34) | THP + C-T (n=26) | P |
---|---|---|---|---|---|---|
UA1 (μmol/L) | 278.32 | 292.18 | 252.18 | 286.26 | 259.08 | 0.15 |
UA2 (μmol/L) | 345.51 | 317.47 | 255.64 | 347.00 | 310.08 | <0.01 |
△UA (μmol/L) | 67.19 | 25.13 | 3.45 | 60.74 | 51.00 | <0.01 |
The data is expressed as an average. Ua1 represents the level of serum uric acid at the first diagnosis; UA2 represents the level of serum uric acid in the last chemotherapy; △UA represents the difference between baseline and last chemotherapy serum uric acid level. EC-T, epirubicin-cyclophosphamide followed by paclitaxel; AC-T, adriamycin-cyclophosphamide followed by paclitaxel; CEF, cyclophosphamide + epirubicin + fluorouracil; TEC, paclitaxel + epirubicin + cyclophosphamide; THP + C-T, pirarubicin-cyclophosphamide followed by paclitaxel.
Independent predictors of high SUA in breast cancer patients receiving chemotherapy
In order to determine the factors leading to changes in serum uric acid in patients with breast cancer, we first conducted a univariate logistic regression analysis for each variable to screen variables (Figure 2). Among the variables considered, age (P=0.001), BMI (P<0.001), menstrual status (P=0.005), AC-T and THP + C-T were significantly correlated with changes of SUA in breast cancer patients (Figure 2). Subsequently, we performed multivariate logistic regression analysis on these five factors to comprehensively analyze the impact of multiple factor interaction on SUA.
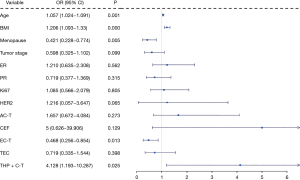
The results of multivariate logistic regression analysis showed that age (P=0.043), BMI (P=0.004), Menopause (P=0.048), EC-T (P=0.045) and THP + C-T (P=0.037) were significantly and independently correlated with the changes of blood uric acid level (Figure 3).
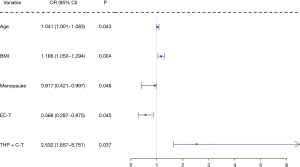
Development and validation of a high SUA prediction nomogram for breast cancer patients receiving chemotherapy
The final prediction model included five independent predictors (age, BMI, menopause, EC-T chemotherapy, THP + C-T chemotherapy), and was developed as a simple and easy-to-use nomogram (Figure 4). The different values of each variable correspond to different positions in the nomogram. A subset of these variables is assigned a count on the point set of the scale, and the respective scores of the points of different variables are calculated and summed to produce a total score. The total score can be converted into the prediction probability of elevated SUA according to the total score axis of the nomogram. The higher the total score, the higher the risk of elevated SUA. However, it should be noted that when using this nomogram, there is a patient’s value on each variable axis. The values of EC-T and THP + C-T cannot be accumulated with each other. Only one of the two chemotherapy regimens can be added to the scores of the other three variables (BMI, age and menopause), Finally, it is possible to predict the increase of SUA induced by chemotherapy in breast cancer patients.
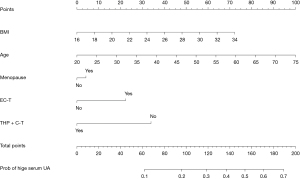
The validation and calibration of the nomogram were performed by 1,000 repeated sampling analysis. The calibration chart of the nomogram is shown in Figure 5, which shows that there is a close consistency between the predicted risk and the actual risk of high SUA, with mean absolute error of the training set is 0.018 (Figure 5A) and the validation set is 0.021 (Figure 5B).
The c-index of the nomogram is 0.706, the corresponding ROC curve and its area under the curve (AUC) in the training cohort is 0.796 (Figure 6A), and the corresponding ROC curve and its AUC in the validation cohort is 0.688 (Figure 6B), which indicates that the prediction model shows relatively good accuracy in estimating the risk of elevated serum uric acid, Individuals with high SUA risk can be distinguished from those without high SUA risk.
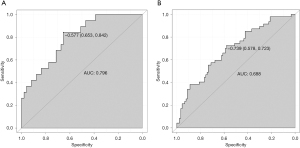
Analysis of decision curve of high SUA nomogram in patients with breast cancer undergoing chemotherapy
Decision curve analysis of high SUA risk prediction nomogram in training set (Figure 7A) and validation set (Figure 7B). None represents the net income when the participant is not considered to have a high SUA, while the All line represents the net income when all participants are considered to have a high SUA. The area between model curves, “None line” and “All line” represent the clinical usefulness of the model. The farther the model curve is from the none line and all line, the better the clinical value of nomogram. Using bootstrap resampling (times =1,000). The results show that for the threshold between 0.2 and 0.9, the maximum benefit of the model is about 0.75. In other words, in a large NB range, the benefit of the model is higher than that of the extreme curve, showing relatively good value.
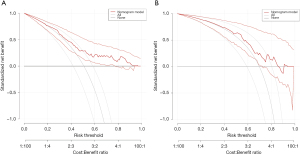
Prognostic value of SUA level in breast cancer patients
The range of serum uric acid concentration was 201 to 570 µmol/L. According to the optimal uric acid cutoff value (UA =355 µmol/L), we divided the samples into Low SUA group (uric acid ≤355 µmol/L) and High SUA group (uric acid >355 µmol/L). During an average follow-up of 80 months (ranging from 0.23 to 79.8 months), 14 patients (26.41%) had bone metastasis, 15 patients (28.30%) had liver metastasis, 15 patients (28.30%) had lung metastasis, 5 patients (9.43%) had brain metastasis, and 4 patients (7.54%) had distant multiple metastases (combined with liver, bone and brain metastases). Among the 206 breast cancer patients, distant metastasis occurred in 32 of 140 (22.86%) patients with a SUA concentration ≤355 µmol/L and in 21 of 66 (31.81%) patients with a SUA concentration >355 µmol/L (Figure 8A). Kaplan-Meier curves showed that there was no significant difference between the high pretreatment uric acid concentration and the low pretreatment uric acid concentration in the occurrence of distant metastasis (DMFS) (P=0.604, log-rank test, Figure 8B).
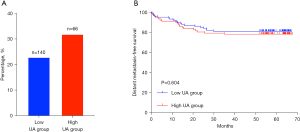
Discussion
This study is the first to detect changes in serum uric acid levels based on different chemotherapy regimens for breast cancer. At the same time, we have also developed and verified a nomogram for predicting the risk of elevated uric acid in breast cancer chemotherapy patients. The nomogram includes age, five independent predictors of BMI, age, menopause, EC-T chemotherapy and THP + C-T chemotherapy making it a practical and easy-to-use tool that can help clinicians when choosing chemotherapy regimens for breast cancer patients, the risk of elevated uric acid can be avoided objectively and relatively correctly.
As a very common metabolite, serum uric acid has many effects on the human body. High levels of serum uric acid are considered harmful to health. Multiple studies have shown that elevated SUA levels may lead to the development of obesity, chronic kidney disease, diabetes, and hypertension (4,16-18), may also cause cancer (10,19,20). High SUA or gout is positively connected to cancer mortality, according to a meta-analysis based on a cohort study that included 24 papers (33 independent investigations) (21). The other is a Mendelian randomization study that analyzed 86,210 individuals from the general population in Copenhagen (22). It shows that the high risk of SUA is related to the high incidence and mortality of cancer, whether it is observed or genetically. In addition, with regard to the mortality rate of specific cancer, Yue et al. (10) conducted a retrospective study involving 443 women, and the results showed that high SUA levels predict low survival rates for breast cancer patients. SUA is closely related to the occurrence and prognosis of cancer, mainly due to the pro-oxidation effect of SUA. In our study, although the overall difference in the occurrence of distant metastasis in breast cancer patients between the high uric acid group and the low uric acid group was not significant (P>0.05), it can be seen that the overall survival curve of the low uric acid group is higher than that of the high uric acid group. This is consistent with the results of previous epidemiological and experimental studies (10). Excessive SUA may affect the survival time of breast cancer patients, suggesting that we should pay attention to reducing SUA levels to improve the prognosis of breast cancer patients.
In order to screen out the independent predictors that affect SUA, a univariate logistic regression analysis was first performed. We observed that the SUA level increased with the increase in BMI, our observations are consistent with previous studies (23,24), that is, obesity is related to hyperuricemia. We also found that age and menopausal status were risk factors for high SUA (25-28), which is consistent with the results of previous studies (29).
As we all know, chemotherapy can increase SUA (30). It is mainly caused by some cytotoxic drugs. Through further exploration, this study found for the first time that the use of EC-T and THP + C-T may be an independent predictor of SUA elevation (P<0.05), the other chemotherapy regimens have no significant impact on SUA (P>0.05), and the comparison shows that Epirubicin has more effect on the increase of SUA than Pirarubicin. Subsequently, we conducted a multivariate logistic regression analysis on the five factors: BMI, age, menopause, EC-T and THP + C-T. The results showed that the five factors have high predictive value for the increase of SUA. On this basis, we built a nomogram forecasting model. The forecasting model is a tool to integrate risk factors and can provide everyone with accurate, objective and personalized risk forecasts.
According to us, this is the first prediction nomogram model for the risk of elevated SUA levels in breast cancer patients undergoing chemotherapy. Based on five variables that are easy to implement and cost effective, it can help clinicians avoid the risk of hyperuricemia in breast cancer patients undergoing chemotherapy, so as to improve their prognosis and quality of life by choosing a more suitable chemotherapy regimen for them. Good discrimination and accuracy were found in both the training cohort (AUC =0.796) and the validation cohort (AUC =0.688), which shows that the model has relatively good prediction ability and can distinguish individuals with high SUA risk from those without high SUA risk.
However, our study has some potential limitations. First, the relatively small sample size is a major limitation. Second, like other retrospective studies, we cannot completely rule out selection bias. Although the main factors affecting SUA were included, this study did not collect information on other factors reported to affect SUA, such as ER and PR. In addition, among many breast cancer chemotherapy regimens, we only discussed the impact of 8 common chemotherapy regimens on SUA, and further research is needed to explore the specific impact of other chemotherapy regimens on breast cancer.
Despite the above limitations, we have confirmed that BMI, age, menopause, EC-T chemotherapy and THP + C-T chemotherapy are independent risk factors for high SUA. At the same time, we have also established a nomogram for high SUA risk prediction to help clinicians Doctors make individualized choice of chemotherapy regimens.
Acknowledgments
Funding: The present study was supported by the National Natural Science Foundation of China (No. 81960542 and No. 81960517), Science and Technology Project of Yunnan Provincial Science and Technology Department (No. 202001AU070053 and No. 202001AU070093), Scientific Research Foundation of Yunnan Education Department (No. 2019J1288 and No. 2020J0198), and Yunnan Health Training Project of High Level Talents (No. H-2019075).
Footnote
Reporting Checklist: The authors have completed the STROBE statement checklist. Available at https://tbcr.amegroups.com/article/view/10.21037/tbcr-21-34/rc
Data Sharing Statement: Available at https://tbcr.amegroups.com/article/view/10.21037/tbcr-21-34/dss
Peer Review File: Available at https://tbcr.amegroups.com/article/view/10.21037/tbcr-21-34/prf
Conflicts of Interest: All authors have completed the ICMJE uniform disclosure form (available at https://tbcr.amegroups.com/article/view/10.21037/tbcr-21-34/coif). The authors have no conflicts of interest to declare.
Ethical Statement:
Open Access Statement: This is an Open Access article distributed in accordance with the Creative Commons Attribution-NonCommercial-NoDerivs 4.0 International License (CC BY-NC-ND 4.0), which permits the non-commercial replication and distribution of the article with the strict proviso that no changes or edits are made and the original work is properly cited (including links to both the formal publication through the relevant DOI and the license). See: https://creativecommons.org/licenses/by-nc-nd/4.0/.
References
- Cree IA, Indave Ruiz BI, Zavadil J, et al. The International Collaboration for Cancer Classification and Research. Int J Cancer 2021;148:560-71. [Crossref] [PubMed]
- Barzaman K, Karami J, Zarei Z, et al. Breast cancer: Biology, biomarkers, and treatments. Int Immunopharmacol 2020;84:106535. [Crossref] [PubMed]
- Wang Q, Wen X, Kong J. Recent Progress on Uric Acid Detection: A Review. Crit Rev Anal Chem 2020;50:359-75. [Crossref] [PubMed]
- Ndrepepa G. Uric acid and cardiovascular disease. Clin Chim Acta 2018;484:150-63. [Crossref] [PubMed]
- Giordano C, Karasik O, King-Morris K, et al. Uric Acid as a Marker of Kidney Disease: Review of the Current Literature. Dis Markers 2015;2015:382918. [Crossref] [PubMed]
- Katsiki N, Dimitriadis GD, Mikhailidis DP. Serum Uric Acid and Diabetes: From Pathophysiology to Cardiovascular Disease. Curr Pharm Des 2021;27:1941-51. [Crossref] [PubMed]
- Sanchez-Lozada LG, Rodriguez-Iturbe B, Kelley EE, et al. Uric Acid and Hypertension: An Update With Recommendations. Am J Hypertens 2020;33:583-94. [Crossref] [PubMed]
- Dalbeth N, Choi HK, Joosten LAB, et al. Gout. Nat Rev Dis Primers 2019;5:69. [Crossref] [PubMed]
- Strasak AM, Rapp K, Hilbe W, et al. The role of serum uric acid as an antioxidant protecting against cancer: prospective study in more than 28 000 older Austrian women. Ann Oncol 2007;18:1893-7. [Crossref] [PubMed]
- Yue CF, Feng PN, Yao ZR, et al. High serum uric acid concentration predicts poor survival in patients with breast cancer. Clin Chim Acta 2017;473:160-5. [Crossref] [PubMed]
- Kühn T, Sookthai D, Graf ME, et al. Albumin, bilirubin, uric acid and cancer risk: results from a prospective population-based study. Br J Cancer 2017;117:1572-9. [Crossref] [PubMed]
- Ben Salem C, Slim R, Fathallah N, et al. Drug-induced hyperuricaemia and gout. Rheumatology (Oxford) 2017;56:679-88. [PubMed]
- Criscuolo M, Fianchi L, Dragonetti G, et al. Tumor lysis syndrome: review of pathogenesis, risk factors and management of a medical emergency. Expert Rev Hematol 2016;9:197-208. [Crossref] [PubMed]
- Calvo Villas JM. Tumour lysis syndrome. Med Clin (Barc) 2019;152:397-404. [Crossref] [PubMed]
- Amin MB, Greene FL, Edge SB, et al. The Eighth Edition AJCC Cancer Staging Manual: Continuing to build a bridge from a population-based to a more "personalized" approach to cancer staging. CA Cancer J Clin 2017;67:93-9.
- Mortada I. Hyperuricemia, Type 2 Diabetes Mellitus, and Hypertension: an Emerging Association. Curr Hypertens Rep 2017;19:69. [Crossref] [PubMed]
- Kuwabara M, Borghi C, Cicero AFG, et al. Elevated serum uric acid increases risks for developing high LDL cholesterol and hypertriglyceridemia: A five-year cohort study in Japan. Int J Cardiol 2018;261:183-8. [Crossref] [PubMed]
- Shani M, Vinker S, Dinour D, et al. High Normal Uric Acid Levels Are Associated with an Increased Risk of Diabetes in Lean, Normoglycemic Healthy Women. J Clin Endocrinol Metab 2016;101:3772-8. [Crossref] [PubMed]
- Stotz M, Szkandera J, Seidel J, et al. Evaluation of uric acid as a prognostic blood-based marker in a large cohort of pancreatic cancer patients. PLoS One 2014;9:e104730. [Crossref] [PubMed]
- Shin HS, Lee HR, Lee DC, et al. Uric acid as a prognostic factor for survival time: a prospective cohort study of terminally ill cancer patients. J Pain Symptom Manage 2006;31:493-501. [Crossref] [PubMed]
- Xie Y, Xu P, Liu K, et al. Hyperuricemia and gout are associated with cancer incidence and mortality: A meta-analysis based on cohort studies. J Cell Physiol 2019;234:14364-76. [Crossref] [PubMed]
- Kobylecki CJ, Afzal S, Nordestgaard BG. Plasma Urate, Cancer Incidence, and All-Cause Mortality: A Mendelian Randomization Study. Clin Chem 2017;63:1151-60. [Crossref] [PubMed]
- Ali N, Perveen R, Rahman S, et al. Prevalence of hyperuricemia and the relationship between serum uric acid and obesity: A study on Bangladeshi adults. PLoS One 2018;13:e0206850. [Crossref] [PubMed]
- Biradar MI, Chiang KM, Yang HC, et al. The causal role of elevated uric acid and waist circumference on the risk of metabolic syndrome components. Int J Obes (Lond) 2020;44:865-74. [Crossref] [PubMed]
- Liu DM, Jiang LD, Gan L, et al. Association between serum uric acid level and body mass index in sex- and age-specific groups in southwestern China. Endocr Pract 2019;25:438-45. [Crossref] [PubMed]
- Konta T, Ichikawa K, Kawasaki R, et al. Association between serum uric acid levels and mortality: a nationwide community-based cohort study. Sci Rep 2020;10:6066. [Crossref] [PubMed]
- MacFarlane LA, Kim SC. Gout: a review of nonmodifiable and modifiable risk factors. Rheum Dis Clin North Am 2014;40:581-604. [Crossref] [PubMed]
- Saag KG, Choi H. Epidemiology, risk factors, and lifestyle modifications for gout. Arthritis Res Ther 2006;8:S2. [Crossref] [PubMed]
- Li L, Zhang Y, Zeng C. Update on the epidemiology, genetics, and therapeutic options of hyperuricemia. Am J Transl Res 2020;12:3167-81. [PubMed]
- Wang J, Fan Y, Cai X, et al. Uric acid preconditioning alleviated doxorubicin induced JNK activation and Cx43 phosphorylation associated cardiotoxicity via activation of AMPK-SHP2 signaling pathway. Ann Transl Med 2020;8:1570. [Crossref] [PubMed]
Cite this article as: Wang Q, Yang D, Mei J, Li S, Xie S, Zhang H, Dai L, Zhu Z, Lv Y, Yang F, Liu D, Guo R, Tang S. The study of correlation between nomogram prediction of uric acid and different chemotherapy regimens in breast cancer patients. Transl Breast Cancer Res 2022;3:14.